Understanding Machine Learning Annotation: A Key to Business Success
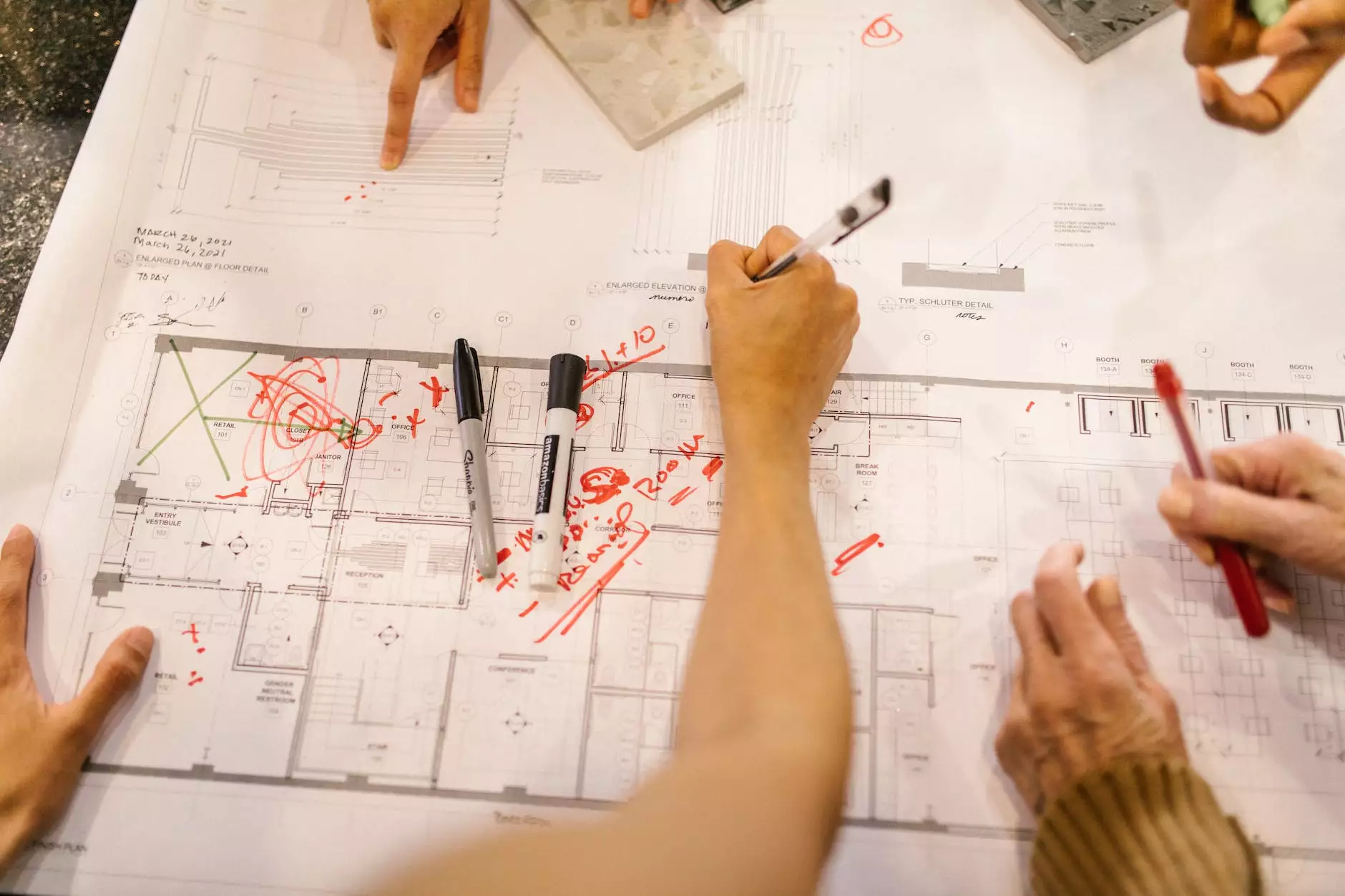
In the modern technological landscape, machine learning has emerged as a game-changer across various industries. At the heart of effective machine learning is data—specifically, the quality of that data. This is where machine learning annotation plays a crucial role. In this article, we will explore what machine learning annotation is, its significance, and how businesses can leverage annotated data to enhance their operations.
What is Machine Learning Annotation?
Machine learning annotation refers to the process of labeling data so that machine learning algorithms can understand and learn from it. This data can come in various forms, including images, text, audio, and video. By providing context through annotation, businesses can train models to recognize patterns, make predictions, and improve decision-making.
Types of Machine Learning Annotation
There are several types of annotations that cater to different forms of data. Understanding these types is crucial for businesses looking to implement machine learning solutions:
- Image Annotation: This includes labeling images with bounding boxes, segmentation masks, or key-point annotations. For example, in self-driving cars, annotated images help the AI recognize pedestrians and obstacles.
- Text Annotation: Text data can be annotated for sentiment analysis, named entity recognition, and classification. For instance, businesses can analyze customer feedback through labeled text to improve services.
- Audio Annotation: This involves transcribing speech or labeling sound events in audio files, crucial for applications like voice recognition software.
- Video Annotation: Annotating video content allows for tracking objects across frames and recognizing activities, which is vital for surveillance systems and automated editing tools.
The Importance of Machine Learning Annotation in Business
For businesses venturing into the world of artificial intelligence, the importance of precise and high-quality machine learning annotation cannot be overstated. Here are several reasons why it is essential:
1. Enhanced Accuracy
Accurate machine learning annotation leads to improved model performance. Well-labeled data enables algorithms to make precise predictions, thus minimizing errors. For businesses, this translates to better insights, which can inform strategy and operational decisions.
2. Accelerated Development Cycles
With an efficient annotation process, businesses can significantly reduce the time needed to develop machine learning models. Quick turnaround times on annotated datasets allow teams to focus on refining their algorithms and deploying solutions faster.
3. Increased Competitiveness
In an age where data drives decisions, having access to high-quality annotated data can set a business apart from its competitors. Companies that understand and implement effective machine learning techniques can better cater to their audience and respond to changing market dynamics.
Implementing Machine Learning Annotation: Best Practices
To fully capitalize on the benefits of machine learning annotation, businesses must adopt certain best practices:
1. Define Clear Annotation Guidelines
Providing clear and concise instructions for annotators ensures consistency and quality across annotated data. Detailed guidelines help reduce ambiguity, which is crucial for maintaining high standards.
2. Choose the Right Tools
Using specialized annotation tools can significantly enhance efficiency. Depending on the types of data you are working with, tools may offer features like collaborative annotation, automated suggestions, and quality control checks.
3. Utilize a Trained Workforce
Having trained annotators who understand the context of the data can lead to better quality annotations. This is particularly important for complex fields such as medical imaging or legal documents.
4. Regular Quality Checks
Implementing a robust verification process is vital to ensure the accuracy of the annotations. Regular audits can help identify inconsistencies and areas for improvement.
The Future of Machine Learning Annotation
As machine learning technology continues to evolve, so do the techniques surrounding machine learning annotation. The future of this field is promising, with advancements that could involve:
- Automation: Increased use of semi-automated annotation tools that combine machine learning with human input, improving evaluation speed and accuracy.
- AI-Assisted Annotation: Techniques that leverage active learning, allowing models to suggest annotations which are then validated by human annotators.
- Integration with Natural Language Processing: Enhanced capabilities for interpreting and analyzing unstructured data, thus enabling richer insights from textual sources.
Case Studies: Success Stories in Machine Learning Annotation
To substantiate the benefits discussed, let’s explore a few real-world applications of machine learning annotation:
1. Autonomous Vehicles
Companies like Tesla and Waymo have invested heavily in machine learning annotation to train their self-driving algorithms. Annotated video and images from various driving environments help these systems detect vehicles, pedestrians, and traffic signs, ensuring safety and reliability.
2. Healthcare Diagnostics
In the healthcare sector, annotated medical images are critical for training machine learning models to recognize diseases. Hospitals and clinics are employing annotated datasets to create software that can analyze x-rays or MRIs, helping radiologists with faster and more accurate diagnoses.
3. E-commerce Personalization
E-commerce giants like Amazon utilize annotated customer data to enhance user experience. By analyzing purchase history and customer reviews through annotated text, they tailor recommendations and marketing strategies, fostering customer loyalty.
Conclusion: Harnessing the Power of Machine Learning Annotation
In conclusion, as the demand for machine learning solutions grows, so does the need for high-quality data annotation. Businesses that prioritize effective machine learning annotation can unlock new opportunities, improve their operations, and enhance customer satisfaction. With the right strategies in place, the potential of machine learning will be fully realized, paving the way for a smarter, more innovative future.
Get Started with Machine Learning Annotation Today!
If you're interested in utilizing machine learning annotation to transform your business operations, look no further than Keymakr. We offer robust annotation services tailored to meet your specific needs, ensuring you receive the high-quality data required for effective machine learning implementation.